Practical Deep Learning for Cloud, Mobile & Edge
** Featured on the official Keras website **
Whether you’re a software engineer aspiring to enter the world of deep learning, a veteran data scientist, or a hobbyist with a simple dream of making the next viral AI app, you might have wondered where to begin. This step-by-step guide teaches you how to build practical deep learning applications for the cloud, mobile, browsers, and edge devices using a hands-on approach. Relying on years of industry experience transforming deep learning research into award-winning applications, Anirudh Koul, Siddha Ganju, and Meher Kasam guide you through the process of converting an idea into something that people in the real world can use.
|
What our readers are saying
Amazon — 4.6 (114) ⭐⭐⭐⭐⭐
Goodreads — 4.71 (38) ⭐⭐⭐⭐⭐
Goodreads — 4.71 (38) ⭐⭐⭐⭐⭐
⭐⭐⭐⭐⭐
I have stepped into a new role at work with the need to become a practitioner of deep learning. I purchased this book to help me optimize some of the machine learning models I've been working with and to help drive knowledge with examples. This book has helped me accelerate this process in many ways. It teaches well with examples that play directly into my scenarios on iOS and Android. It has been useful already in several ways -- in particular, it has helped me to understand how to deal with an overfitting problem I have been dealing with. — Yevgeniy on Amazon |
⭐⭐⭐⭐⭐
Being a CS major, I always wanted to get myself familiar with Machine Learning and AI. But I was confused about what resource would be the best to get started. The "learn by doing projects" approach of this book never made me feel like I was just reading a book. I recently won a hackathon by using the practical knowledge I gained from this. — Arman Sidhu on Goodreads |
⭐⭐⭐⭐⭐
Right from the outset, the friendly tone of this book yields a free flowing, friendly funnel to the advanced concepts the reader is trying to learn. With this friendly approach, the intimidation factor of difficult concepts like Deep Neural Networks become a little bit more human. It also helps that the examples they have selected, from the references to the HBO series 'Silicon Valley' and trying out 'Shazam' for food create a fun learning experience while diving in sufficient depth for any new ML engineer. I learnt a lot from this book, and intend to use this knowledge in my day-to-day. It also helps that some of the authors have done this in practice in the real world with groundbreaking apps like Seeing AI. Great writing and strongly recommended! — Mutant Programmer on Amazon |
⭐⭐⭐⭐⭐
As someone who tried to learn Deep Learning through several online courses, one of the biggest issues was wading through too much theory and the inability to apply the concepts outside the course. However, Practical Deep Learning was extremely refreshing in several aspects - its structure, applicability, intelligibility, and empathy. The learner can start applying the concepts from the very beginning with the help of the GitHub repo which makes one think outside the theory in the practical realm as soon as they kick-off. And I found this book to be very unlike any technical book that I ever read. Explaining highly complex concepts with real-life analogies and a sense of humor clearly shows deep empathy by the authors for the readers who can be complete novices to an expert. This book is one of the engaging technical writing out there and I found it so helpful that after the first 3 chapters I ordered another one as a gift for a friend. — Amazon Customer on Amazon |
Table of contents
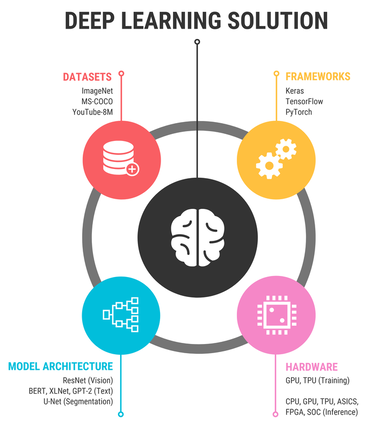
Chapter 1 - Exploring the Landscape of Artificial Intelligence
We take a tour of this evolving landscape, from 1950s till today, and analyze the ingredients that make for a perfect deep learning recipe, get familiar with common AI terminology and datasets, and take a peek into the world of responsible AI.
Chapter 2 - What’s in the Picture: Image Classification with Keras
We delve into the world of image classification in a mere five lines of Keras code. We then learn what neural networks are paying attention to while making predictions by overlaying heatmaps on videos.
Chapter 3 - Cats versus Dogs: Transfer Learning in 30 Lines with Keras
We use transfer learning to reuse a previously trained network on a new custom classification task to get near state-of-the-art accuracy in a matter of minutes. We then slice and dice the results to understand how well is it classifying. Along the way, we build a common machine learning pipeline, which is repurposed throughout the book.
Chapter 4 - Building a Reverse Image Search Engine: Understanding Embeddings
Like Google Reverse Image Search, we explore how one can use embeddings—a contextual representation of an image to find similar images in under ten lines. And then the fun starts when we explore different strategies and algorithms to speed this up at scale, from thousands to several million images, and making them searchable in microseconds.
We take a tour of this evolving landscape, from 1950s till today, and analyze the ingredients that make for a perfect deep learning recipe, get familiar with common AI terminology and datasets, and take a peek into the world of responsible AI.
Chapter 2 - What’s in the Picture: Image Classification with Keras
We delve into the world of image classification in a mere five lines of Keras code. We then learn what neural networks are paying attention to while making predictions by overlaying heatmaps on videos.
Chapter 3 - Cats versus Dogs: Transfer Learning in 30 Lines with Keras
We use transfer learning to reuse a previously trained network on a new custom classification task to get near state-of-the-art accuracy in a matter of minutes. We then slice and dice the results to understand how well is it classifying. Along the way, we build a common machine learning pipeline, which is repurposed throughout the book.
Chapter 4 - Building a Reverse Image Search Engine: Understanding Embeddings
Like Google Reverse Image Search, we explore how one can use embeddings—a contextual representation of an image to find similar images in under ten lines. And then the fun starts when we explore different strategies and algorithms to speed this up at scale, from thousands to several million images, and making them searchable in microseconds.
Chapter 5 - From Novice to Master Predictor: Maximizing Convolutional Neural Network Accuracy
We explore strategies to maximize the accuracy that our classifier can achieve, with the help of a range of tools including TensorBoard, What-If Tool, tf-explain, TensorFlow Datasets, AutoKeras, AutoAugment. Along the way, we conduct experiments to develop an intuition of what parameters might or might not work for your AI task.
Chapter 6 - Maximizing Speed and Performance of TensorFlow: A Handy Checklist
We take the speed of training and inference into hyperdrive by going through a checklist (downloadable here) of 30 tricks to reduce as many inefficiencies as possible and maximize the value of your current hardware.
Chapter 7 - Practical Tools, Tips, and Tricks
We diversify our practical skills in a variety of topics and tools, ranging from installation, data collection, experiment management, visualizations, keeping track of the state-of-the-art in research all the way to exploring further avenues for building the theoretical foundations of deep learning.
We explore strategies to maximize the accuracy that our classifier can achieve, with the help of a range of tools including TensorBoard, What-If Tool, tf-explain, TensorFlow Datasets, AutoKeras, AutoAugment. Along the way, we conduct experiments to develop an intuition of what parameters might or might not work for your AI task.
Chapter 6 - Maximizing Speed and Performance of TensorFlow: A Handy Checklist
We take the speed of training and inference into hyperdrive by going through a checklist (downloadable here) of 30 tricks to reduce as many inefficiencies as possible and maximize the value of your current hardware.
Chapter 7 - Practical Tools, Tips, and Tricks
We diversify our practical skills in a variety of topics and tools, ranging from installation, data collection, experiment management, visualizations, keeping track of the state-of-the-art in research all the way to exploring further avenues for building the theoretical foundations of deep learning.
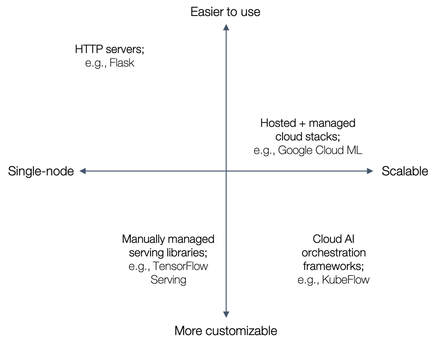
Chapter 8 - Cloud APIs for Computer Vision: Up and Running in 15 Minutes
Work smart, not hard. We utilize the power of cloud AI platforms from Google, Microsoft, Amazon, IBM and Clarifai in under 15 minutes. For tasks not solved with existing APIs, we then use custom classification services to train classifiers without coding. And then we pit them against each other in an open benchmark, you might be surprised who won.
Chapter 9 - Scalable Inference Serving on Cloud with TensorFlow Serving and KubeFlow
We take our custom trained model to the cloud/on-premises to scalably serve from tens to millions of requests. We explore Flask, Google Cloud ML Engine, TensorFlow Serving, and KubeFlow, showcasing the effort, scenario, and cost-benefit analysis.
Chapter 10 - AI in the Browser with TensorFlow.js and ml5.js
Every single individual who uses a computer or a smartphone uniformly has access to one software program—their browser. Reach all those users with browser-based deep learning libraries including TensorFlow.js and ml5.js. Guest author Zaid Alyafeai walks us through techniques and tasks such as body pose estimation, generative adversarial networks (GANs), image-to-image translation with Pix2Pix and more, running not on a server but in the browser itself.
Work smart, not hard. We utilize the power of cloud AI platforms from Google, Microsoft, Amazon, IBM and Clarifai in under 15 minutes. For tasks not solved with existing APIs, we then use custom classification services to train classifiers without coding. And then we pit them against each other in an open benchmark, you might be surprised who won.
Chapter 9 - Scalable Inference Serving on Cloud with TensorFlow Serving and KubeFlow
We take our custom trained model to the cloud/on-premises to scalably serve from tens to millions of requests. We explore Flask, Google Cloud ML Engine, TensorFlow Serving, and KubeFlow, showcasing the effort, scenario, and cost-benefit analysis.
Chapter 10 - AI in the Browser with TensorFlow.js and ml5.js
Every single individual who uses a computer or a smartphone uniformly has access to one software program—their browser. Reach all those users with browser-based deep learning libraries including TensorFlow.js and ml5.js. Guest author Zaid Alyafeai walks us through techniques and tasks such as body pose estimation, generative adversarial networks (GANs), image-to-image translation with Pix2Pix and more, running not on a server but in the browser itself.
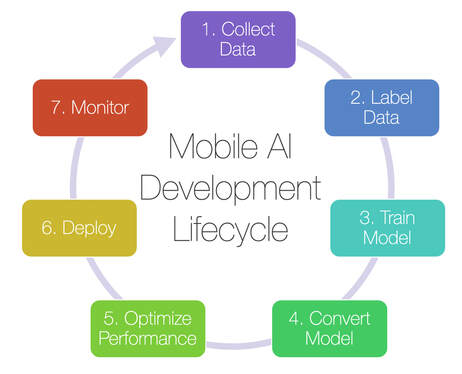
Chapter 11 - Real-Time Object Classification on iOS with Core ML
We explore the landscape of deep learning on mobile, with a sharp focus on the Apple ecosystem with Core ML. We benchmark models on different iPhones, investigate strategies to reduce app size and energy impact, dynamic model deployment, training on device, and how professional apps are built.
Chapter 12 - Not Hotdog on iOS with Core ML and Create ML
Silicon Valley’s Not Hotdog app (from HBO) is considered the “Hello World” of mobile AI, so we pay tribute by building a real-time version in not one, not two, but three different ways.
We explore the landscape of deep learning on mobile, with a sharp focus on the Apple ecosystem with Core ML. We benchmark models on different iPhones, investigate strategies to reduce app size and energy impact, dynamic model deployment, training on device, and how professional apps are built.
Chapter 12 - Not Hotdog on iOS with Core ML and Create ML
Silicon Valley’s Not Hotdog app (from HBO) is considered the “Hello World” of mobile AI, so we pay tribute by building a real-time version in not one, not two, but three different ways.
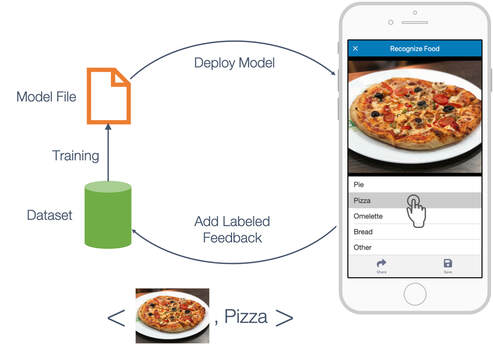
Chapter 13 - Shazam for Food: Developing Android Apps with TensorFlow Lite and ML Kit
We bring AI to Android with the help of TensorFlow Lite. We then look at cross-platform development using ML Kit (which is built on top of TensorFlow Lite) and Fritz to explore the end-to-end development life cycle for building a self-improving AI app. Along the way we look at model versioning, A/B testing, measuring success, dynamic updates, model optimization, and other topics.
Chapter 14 - Building the Purrfect Cat Locator App with TensorFlow Object Detection API
We explore four different methods for locating the position of objects within images. We take a look at the evolution of object detection over the years, and analyze the tradeoffs between speed and accuracy. This builds the base for case studies such as crowd counting, face detection, and autonomous cars.
We bring AI to Android with the help of TensorFlow Lite. We then look at cross-platform development using ML Kit (which is built on top of TensorFlow Lite) and Fritz to explore the end-to-end development life cycle for building a self-improving AI app. Along the way we look at model versioning, A/B testing, measuring success, dynamic updates, model optimization, and other topics.
Chapter 14 - Building the Purrfect Cat Locator App with TensorFlow Object Detection API
We explore four different methods for locating the position of objects within images. We take a look at the evolution of object detection over the years, and analyze the tradeoffs between speed and accuracy. This builds the base for case studies such as crowd counting, face detection, and autonomous cars.
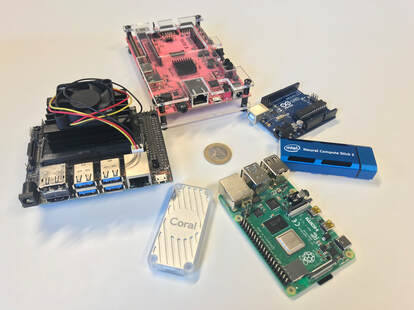
Chapter 15 - Becoming a Maker: Exploring Embedded AI at the Edge
Guest author Sam Sterckval brings deep learning to low-power devices as he showcases a range of AI-capable edge devices with varying processing power and cost including Raspberry Pi, NVIDIA Jetson Nano, Google Coral, Intel Movidius, PYNQ-Z2 FPGA, opening the doors for robotics and maker projects.
Chapter 16 - Simulating a Self-Driving Car using End-to-End Deep Learning with Keras
Using the photorealistic simulation environment of Microsoft AirSim, guest authors Aditya Sharma and Mitchell Spryn guide us in training a virtual car by driving it first within the environment and then teaching an AI model to replicate its behavior. Along the way, this chapter covers a number of concepts that are applicable in the autonomous car industry.
Chapter 17 - Building an Autonomous Car in Under an Hour: Reinforcement Learning with AWS DeepRacer
Moving from the virtual to the physical world, guest author Sunil Mallya showcases how AWS DeepRacer, a miniature car, can be assembled, trained and raced in under an hour. And with the help of reinforcement learning, the car learns to drive on its own, penalizing mistakes and maximizing success. We learn how to apply this knowledge to races from the Olympics of AI Driving to RoboRace (using full-sized autonomous cars).
Guest author Sam Sterckval brings deep learning to low-power devices as he showcases a range of AI-capable edge devices with varying processing power and cost including Raspberry Pi, NVIDIA Jetson Nano, Google Coral, Intel Movidius, PYNQ-Z2 FPGA, opening the doors for robotics and maker projects.
Chapter 16 - Simulating a Self-Driving Car using End-to-End Deep Learning with Keras
Using the photorealistic simulation environment of Microsoft AirSim, guest authors Aditya Sharma and Mitchell Spryn guide us in training a virtual car by driving it first within the environment and then teaching an AI model to replicate its behavior. Along the way, this chapter covers a number of concepts that are applicable in the autonomous car industry.
Chapter 17 - Building an Autonomous Car in Under an Hour: Reinforcement Learning with AWS DeepRacer
Moving from the virtual to the physical world, guest author Sunil Mallya showcases how AWS DeepRacer, a miniature car, can be assembled, trained and raced in under an hour. And with the help of reinforcement learning, the car learns to drive on its own, penalizing mistakes and maximizing success. We learn how to apply this knowledge to races from the Olympics of AI Driving to RoboRace (using full-sized autonomous cars).